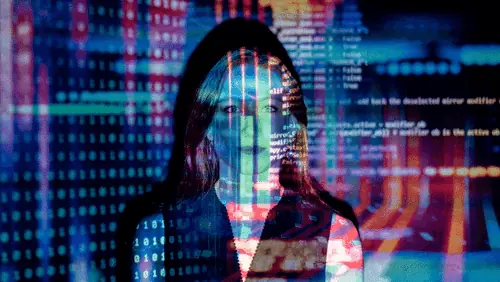
Data leaks hurt consumers. Though the true number of breaches and compromised data remains unknown, those we know of have compromised billions of records--including highly sensitive customer data. Even the largest corporations with the most competitive security teams have failed to prevent data leaks. Until this is properly addressed, consumers cannot trust enterprises to keep their information safe and will continue to see the private information they share with enterprises dangerously exposed, stolen, and shared.
Mounting public concern over how enterprises interact with our personal information has led to the development of laws and regulations, such as the General Data Protection Regulation (GDPR), and the California Consumer Privacy Act (CCPA). Personal data now officially requires special handling and care to meet today’s compliance standards and consumer expectations. This post will discuss one of the most effective techniques to mitigate and reduce the risk of compromised consumer data.
Data Pseudonymization and Data De-Identification
Personal data de-identification is the process of removing identifiers from a data set to prevent any possibility of linking individuals to its information. When de-identification is applied in a way that makes it impossible to link it back to individuals, to re-identify the data, the data is considered anonymized.
Today’s business analysts and data scientists require access to data. However, due to lacking security awareness and problematic infrastructure, direct access introduces unacceptable risk--especially if the data-in-use is subsequently copied outside of controlled systems. Applying the best practice of anonymization or pseudonymization at the very least dramatically reduces these risks.
What is Pseudonymization?
Anonymized data sets are out of the scope of privacy regulations like GDPR. However, full anonymization can disrupt many legitimate data uses that require identifiers (e.g., your bank probably needs to know who you are as a user). GDPR proposes pseudonymization as a practical alternative for reducing the risk of data exposure, relieving compliance obligations, and maintaining optimal data utilization:
‘pseudonymisation’ means the processing of personal data in such a manner that the personal data can no longer be attributed to a specific data subject without the use of additional information, provided that such additional information is kept separately and is subject to technical and organisational measures to ensure that the personal data are not attributed to an identified or identifiable natural person (link)
Pseudonymization is the process of replacing all sensitive identifiers with pseudonyms/aliases/tokens in a specific data set (e.g., a table in a database or a CSV file). The original identifiers have to be kept securely elsewhere. C’est tout. Unfortunately, it’s much more complicated to execute. Building systems that work with pseudonymized data requires a whole lot of designing from the get-go. This is incredibly difficult to do--so much so that GDPR can only recommend it due to unenforceability.
What is Tokenization?
Tokenization is the process of substituting a sensitive data element with a non-sensitive equivalent, referred to as a token, that has no exploitable meaning or value. The token is a reference that maps back to the sensitive data through a tokenization system.
Data Anonymization Techniques
Pseudonymization is typically implemented by using a tokenization technique (see below). Note that, like anonymization, pseudonymized data cannot be linked to a person's specific identity on its own. However, unlike anonymization, it is possible to re-identify pseudonymized data using the additional piece of information kept secure elsewhere. Effectively, when systems require the original plaintext identifiers, they can still translate the pseudonyms back.
Example of an original table (with PIIs of emails and SSNs):
Okay, if you get the concept and want to protect PII, you can sign up for a free account, set up a cloud hosted vault, and tokenize data using our APIs in 2 minutes from now.
Social Security Number is now tokenized:
Both emails and SSNs are tokenized, and the table is now pseudonymized using PII tokenization.
* Email addresses are tokenized using a format-preserving tokenization mechanism.
* This table is now either anonymized or pseudonymized depending on whether it's possible to restore its original email or SSN values.
This is a 1:1 translation (tokens) table matching tokens to the original
* Authorizing, auditing, and monitoring access to this table are critical for preserving the anonymity of the users in the original table.
Data Tokenization Explained
Tokenization is the process of substituting a single piece of sensitive information with non-sensitive information. The non-sensitive substitute information is called a token. It can be created using cryptography, a hash function, or a randomly generated index identifier and used to redact the original sensitive information. For example, tokenizing sensitive information like PII or credit card numbers is important when logging them into a file.
The operation of detokenization, or translating a token to its corresponding data, should only be done on a need-to-know basis (using the right permissions).To support various use cases, tokenization engines that are used for pseudonymization must account for the following possible requirements:
- Format-preserving tokens: These tokens preserve the format of the original data. They are often required in situations with strict storage formatting, such as when changing the database scheme is impossible.
- Deterministic vs. unique tokens: This refers to whether the same value is always mapped to the same token or if each value is mapped to its own unique token. Deterministic tokenization enables users to lookup exact matches and performs joins between tokenized directly on the columns of a pseudonymized dataset. However, this means that it still leaks some information about original identifiers and consequently reduces the data protection provided by the tokenization engine. For example, it exposes the fact that two different identifiers are the same and, using statistical methods, can be used to reveal the original data.
- Ephemeral tokens: These tokens are valid for a limited amount of time. Their expiry limits exposure. They are often used for regular tasks, for example, identifiers exported on a nightly job from a transactional system to an analytical data pipeline.
- Querying the data: The ability to perform lookups on the tokenized data, such as searching for all emails with the domain “@example.com” in the example above.
- Efficiently updating/deleting identifiers: The process of only updating referenced identity (e.g., inside the translation/tokens table) instead of updating multiple tables holding a specific token while keeping the token as-is.
Tokenization engines for pseudonymization can be implemented in two main ways: based on a translation table or based on encryption. Table-based tokenization maintains a mapping between identifiers to randomly generated tokens in a table that is stored in a centralized location. Encryption-based tokenization uses a cryptographic algorithm and corresponding key to translate identifiers to tokens and vice versa. Both the table and the key must be secured and stored separately from the original database.
To Summarize
The following table summarizes important considerations for both approaches:
Sometimes it’s hard to tell which model is better for you. In such cases, it’s best determined by the use case at hand. Typically, it’s best to use a table when you need more searchability over the data. If you need more performance and data security, but less searchability, then encryption may be best. Sometimes, if you know the architecture and how it uses the data, you can roll your own solution to play around with these characteristics.
Whatever it is you choose to do around pseudonymization, you'll have to go through building the tokenization engine first. It takes time and effort, not to mention expertise to build something that is production-grade that can easily scale for thousands of requests per second (RPS). Instead, you can sign up for a free Vault trial, and check out our rich tokenization APIs.
It all begins with the cloud, where applications are accessible to everyone. Therefore, a user or an attacker makes no difference per se. Technically, encrypting all data at rest and in transit might seem like a comprehensive approach, but these methods are not enough anymore. For cloud hosted applications, data-at-rest encryption does not provide the coverage one might expect.
Senior Product Owner